Previous | Next |
Kang Li1, Xiaoping Qian2, Caitlin Martin3, and Wei Sun3
1Illinois Institute of Technology   2University of Wisconsin-Madison   3Georgia Institute of Technology
IDETC 2014 Prakash Krishnaswami CAPPD Best Paper Award
Demo
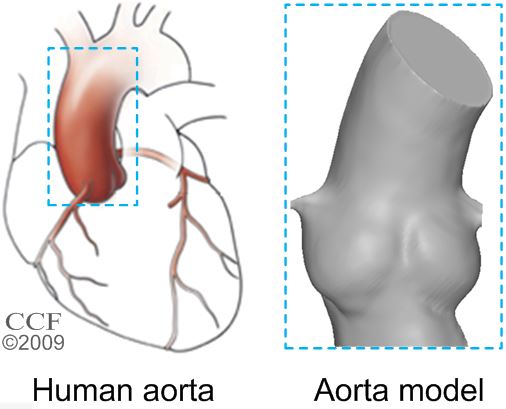
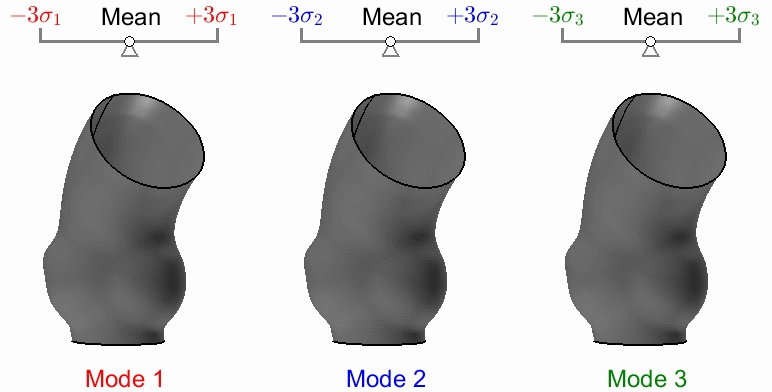
Abstract
Patient-specific computational study of aortic disease provides a powerful means for diagnosis and pre-operative planning. However, creating patient-specific computational models can be time-consuming due to the fact that anatomical geometries extracted from clinical imaging data are often incomplete and noisy. This paper presents an approach for constructing statistical shape models (SSMs) for aortic surfaces with the eventual goal of mapping the mean aortic geometrioes to raw surface data obtained from the clinical images for each new patient so that patient-specific models can be automatically constructed. The input aortic models in this study come in the form of triangle meshes generated from CT scans on 6 patients. Statistical models with modes that characterize the variation pattern are found after optimizing the group-wise correspondence across the aorta training set. We use the direct reparameterization approach to efficiently manipulate shape correspondence. We use B-spline based differentiable shape representation for the training set and use the adjoint method for deriving analytical gradients in a gradient based approach for manipulating the shape correspondence to minimize the description length of the resulting SSM. Our numerical result shows that the evaluation measures of the optimized statistical model have been significantly enhanced.